Surveying graduate Vaughn Golding has published research on using AI to help detect cracks in concrete that could be a game changer.
Golding’s research, conducted while he was studying a Bachelor of Spatial Science (Honours) at the University of Southern Queensland, may point to new ways to detect faults in concrete infrastructure. Golding’s research has been featured in the international academic journal, Sustainability.
“Infrastructure, such as buildings, bridges, and pavements, needs to be examined periodically to maintain reliability and structural health,” says Golding.
“At the moment, experienced inspectors need to examine the concrete in person. Sometimes their equipment is as simple as a ruler and a cherry picker. Inspection times are long, and the results rely on the inspectors’ empirical and subjective knowledge.
“This lengthy process can result in delays, and of course that can further compromise the infrastructure’s structural integrity.”
“Optical methods, using basic algorithms that detect edges, were only 60 or 70% accurate. Obviously there was room for improvement,” says Golding.
Golding’s original aim was to try to improve the measurement of crack depth using imagery. “I started looking into thermal imaging,” he says. “But then COVID hit, and I couldn’t get out and about to work in the field.”
Looking for a new avenue of research, Golding started to delve into the processes behind automatic ways cracks in concrete infrastructure are detected using technology such as drones.
“Essentially, automated crack detection comes down to two main methods – deep learning and image processing,” he says.
“There has been a lot of research into image-based automated crack detention, but crack enhancement in automated machine learning or deep learning detection is a relatively unexplored area.”
Preprocessing images for better AI results
Golding continues, “I wanted to explore how preprocessing images ahead of deep learning techniques could overcome shortcomings. It’s essentially a hybrid of older techniques like optical methods and modern practices of utilising artificial intelligence.
“To do this, we put a dataset of 40,000 images through its paces, investigating the impacts of image processing techniques such as greyscale, thresholding and edge detection.”
“Our results uncovered interesting characteristics, such as the benefits of greyscale images to improve crack detection training when integrating images into a machine learning model.”
Golding warns that you have to choose the right images for training. “We had to make sure our data was as balanced as possible. Because the AI is aiming for a certain level of accuracy, if your data set is unbalanced, the AI will return false positives because it figures out it can be 80% accurate by simply identifying everything as a crack.”
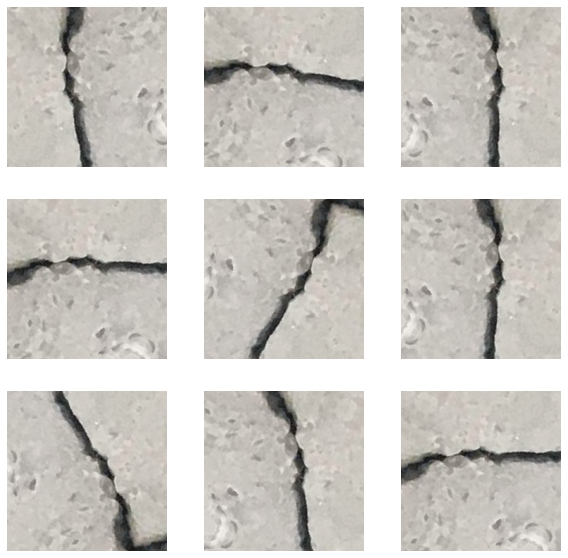
Improvements in sustainability
Having shown that greyscale images perform just as well as RGB images, Golding highlights another benefit of greyscale images – they contain less data, and therefore take less time to process.
“The current thinking is that the best way to train an AI is to give it more information,” says Golding. “But after a while it becomes this massive beast. And with RGB images, because there’s so much information there, it chews through an enormous amount of energy and it takes a long time.”
“By showing that greyscale images work just as well as RGB images, we can now use a third of the amount of data; making it faster and using less energy while keeping the accuracy is a great result.”
What’s next?
Pavement, metal and brick could all be future targets for AI-powered crack detection. But, says Golding, that would take more work.
“My research only looks at concrete, and that’s all I tested it on. AI works best when it’s very specialised,” he says. “I can imagine a future where we have a separate AI that detects the surface that is being measured, and then applies a specialised algorithm depending on the material that it’s dealing with.”
For Golding, though, research is taking a back seat. He’s now concentrating on starting his career – a full-time role with a Townsville surveying firm.
You can read Crack Detection in Concrete Structures Using Deep Learning via MDPI’s Sustainability journal.