The first image of a black hole is the culmination of years of research and experimentation with computational imaging and modelling to stitch together petabytes of telescope data into a single image.
Like the ring of fire it resembles, the world’s first image of a black hole is now burned into the mind’s eye of people around the world.
But another image is making the rounds, and it’s one that perfectly captures the dedication and behind-the-scenes work of the people who make world-changing events like this possible.
The photo is of the moment electrical engineer and computer scientist Dr Katie Bouman gets her first glimpse of what her algorithm made possible. Here, the event horizon of the black hole at the centre of the M87 galaxy plays second fiddle to Bouman. Her expression is one of barely contained glee as she watches the image take shape on her computer screen.
“Watching in disbelief as the first image I ever made of a black hole was in the process of being reconstructed. I’m so excited that we finally get to share what we have been working on for the past year!” she said in a post celebrating the event.
Capturing the image was a mammoth effort made possible by the Event Horizon Telescope (EHT), an international collaboration between engineers, scientists and researchers. For four days in April 2017, the EHT peered across interstellar space to capture data on the M87 black hole. And then last June, members of the EHT project gathered in Cambridge, Massachusetts to see if they could combine this “mountain of data” into a single image.
As one of the engineers working on the project, Bouman and her colleagues wrote the algorithm that stitched together the 4 to 5 petabytes of data to deliver this world-first.
Into the unknown
Bouman received her undergraduate degree in electrical engineering from the University of Michigan in 2011. She went on to receive a PhD in electrical engineering and computer science from the Massachusetts Institute of Technology (MIT) in 2017, but had started working on the EHT team while she was still a graduate student.
Her area of expertise is computational imaging, which focuses on pushing the boundaries of imaging technology through tight integration between algorithms and sensors. This field makes it possible to observe phenomena previously difficult or impossible to measure with traditional approaches — sound familiar?
The black hole at the centre of M87 is so far away (55 million lightyears) and so large (it would take 2.98 million Earths lined up in a row to span its width) that it took a global network of eight telescopes located in Hawai’i, Chile, Mexico, Spain, Arizona and Antarctica to capture it. Together, these telescopes comprise the EHT.
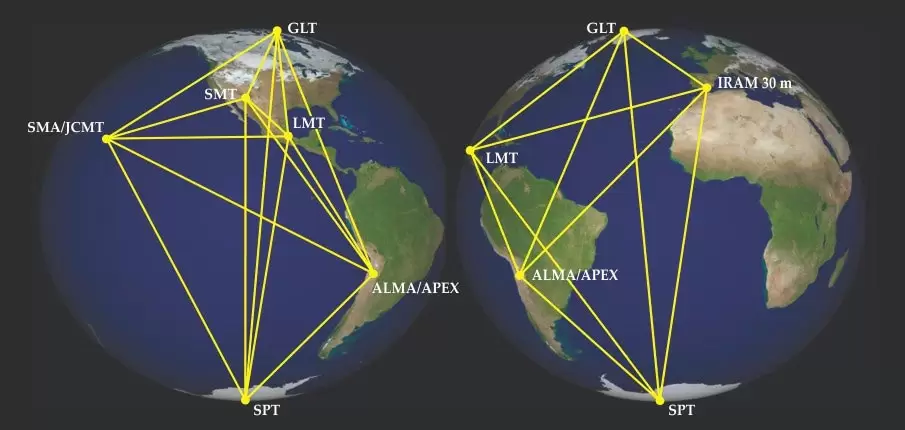
The EHT captured so much data it couldn’t be sent over the internet; instead, a mass of hard drives weighing half a ton had to be flown to two processing centres to be merged.
Left: MIT computer scientist Katie Bouman w/stacks of hard drives of black hole image data.
Right: MIT computer scientist Margaret Hamilton w/the code she wrote that helped put a man on the moon.
(image credit @floragraham)#EHTblackhole #BlackHoleDay #BlackHole pic.twitter.com/Iv5PIc8IYd
— MIT CSAIL (@MIT_CSAIL) April 10, 2019
Seeing the “unseeable”
Engineers and scientists from MIT’s Computer Science and Artificial Intelligence Laboratory (CSAIL), the Harvard-Smithsonian Center for Astrophysics and the MIT Haystack Observatory were tasked with creating the algorithms that would crunch the “really sparse, really noisy data” into a single, profound image.
The first iterations of these algorithms were created and tested in 2016. At the time, researchers from the three institutions were looking at how to use radio telescopes to capture images of black holes. Radio waves can pierce through galactic dust, but they also require very large antenna dishes.
“We would need a telescope with a 10,000 kilometer diameter, which is not practical, because the diameter of Earth is not even 13,000 kilometers,” Bouman said at the time.
The eight sites in the EHT network approximate a 10,000 km-wide antenna and use a technique called very long baseline interferometry imaging (VLBI), which coordinates measurements from telescopes around the world, observing at a wavelength of 1.33 mm with an angular resolution of 20 micro-arcseconds. According to the EHT website, this is “enough to read a newspaper in New York from a sidewalk cafe in Paris”.
However, this still leaves large gaps in the data, and the distance between the telescopes and factors like atmospheric conditions exaggerate the differences in signals, which can prevent accurate imaging.
To fill in these gaps, Bouman developed an algorithm called CHIRP, or Continuous High-Resolution Image Reconstruction using Patch priors. Normally, algorithms make sense of astronomical interferometric data by assuming an image is a collection of points of light. It tries to find points where brightness and location best correspond to the data, and then it blurs together bright points near each other to create some continuity in the image.
CHIRP uses a more complex algorithmic model to preserve the continuity of the image and make it more reliable. Bouman also used machine learning to identify visual patterns that tend to recur in 64-pixel patches to refine the algorithm’s image reconstruction capabilities.
Using VLBI can return an infinite number of possible images that explain the data. Resolving this was another challenge for Bouman and her colleagues.
Data from the EHT was analysed by four separate teams to verify the findings. Two teams relied on a tried-and-true computational imaging method called CLEAN, while the other two used a newer technique called regularised maximum likelihood (RML), which had been honed by Bouman, astrophysicist Andrew Chael from Harvard (who has also been celebrated for this work), and colleagues for the needs of the black hole imaging project.
The four groups were isolated from each other for a week while their images took shape. Although the resulting four images weren’t identical, they all shared a fundamental feature: a roughly 40 micro-arcseconds photon ring surrounding a black hole.
“Even though we had worked on this for years, I don’t think any of us expected we would get a ring that easily. We just expected a blob,” Bouman said.
Back in 2017, she gave a more detailed TED Talk explaining how an image of a black hole could be captured in the next couple of years – which has now come true.
Thousands of simulations were also run to predict what EHT would see and accounted for slightly different values for properties such as plasma temperature, spin and magnetic flux. The modelled black holes look similar to the real one.
The image was created last June, but the full suite of images and research was released last week in a series of six papers in a special issue of The Astrophysical Journal Letters.
Filling the void
Producing the first image of a black hole is a career highlight, but the EHT team already has plans in place to build on this finding. The next observation run for the M87 black hole is scheduled for Spring 2020 using 11 telescopes. The EHT team also plans to turn its eyes to Sagittarius A*, the black hole at the centre of our Milky Way.
In an interview with Time, Bouman said her passion is “coming up with ways to see or measure things that are invisible”. To this end, she will continue to work in fields like signal processing, computer vision, machine learning and physics to further improve imaging technology to help the dark corners of the universe come into focus.
Bouman will join Caltech’s engineering and applied sciences division as an assistant professor in the Department of Computing and Mathematical Sciences in June. To continue her pursuit of ‘visualising the unseeable’, she plans to develop technology that can look around corners by analysing tiny shadows and determining the material properties of objects in videos by measuring tiny motions that are invisible to the naked eye.