Current processes of sorting through construction and demolition waste for recycling purposes are inefficient, costly and hazardous to health. This PhD student is harnessing the power of AI and robotics to revolutionise construction waste recycling in Australia.
The construction industry uses an exorbitant amount of resources, producing a significant proportion of the nation’s waste.
Building construction activities alone account for around 60 per cent of raw material consumption, and are responsible for 40 per cent of solid waste disposed of in landfills across all industries, according to Monash University student Diani Sirimewan, who is undertaking a PhD in civil engineering.
This has both environmental and economic impacts, including a shortage of landfills to accommodate the significant volume of waste.
“There’s a cost for transporting waste from site to landfills, with contractors needing to pay a levy,” Sirimewan told create. “While costs vary across Australia, the charge is around $130 per tonne in Victoria.”
Then there are costs and timeliness associated with sorting through the waste to pick out recyclable materials – made all the more difficult with current labour shortages.
“After construction and demolition waste is collected from different sites, it’s often laid down on the floor, with labourers manually picking out valuable materials such as timber,” Sirimewan explained.
“Because the labourers are manually sorting contaminated waste, there are also health and safety concerns, as well as the need to move heavy and bulky materials.”
With these impacts and inefficiencies in mind, Sirimewan had the idea to use images collected from Melbourne construction sites to train deep learning models and AI to recognise recyclable and contaminated materials.
Creating a skip bin master
Significant research has been conducted into employing robotic technology to sort through domestic waste. But limited datasets, technology and knowledge for application to the construction industry have meant this has been an unexplored frontier, Sirimewan said.
To train the AI to sort through dense construction waste to categorise valuable recyclables such as concrete aggregates, timber, hard plastic, cardboard or metalglass, she collected hundreds of images of skip bins containing mixed construction materials – annotating them using a Python-based automation tool.
“Polygons were drawn around objects such as concrete, which were assigned a colour,” Sirimewan said. “Then I annotated all my data sets, which were used to train the deep learning models to recognise the composition of mixed construction waste.”
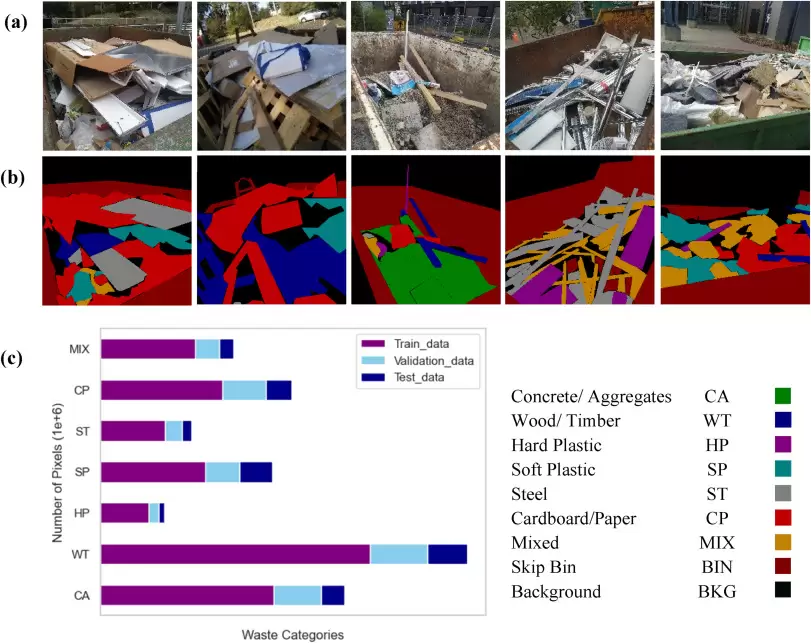
A semantic segmentation approach was then used, where each pixel of an image was assigned to a specific colour, so that it can be distinguished from other objects.
“If you feed an unseen image to the model, the model recognises the composition of it, differentiating waste materials such as concrete, timber or metal, for example,” Sirimewan said. “This is the basic model that can be used for the implementation of robots to sort waste.”
Detecting hazardous contaminants
Recognising recyclable materials is just one capability of the technology. The deep learning models can also be trained to detect small particles, allowing harmful continents to be identified.
This process required careful annotation to ensure utmost accuracy, according to Sirimewan.
“If there are any contaminants in the waste, we need to annotate them specifically,” she said.
“For example, if there’s an asbestos particle we would label it as such, so then the model can recognise asbestos in mixed construction waste.”
Once construction materials have been recycled, the next stage of the research entails improving anomaly detection for quality assurance of recycled materials.
“With anomaly detection, any contaminate particles can be recognised in recycled materials before sending them to a customer. Recycled materials go by on a conveyor belt, so any contaminants inside the recycled materials can be identified.”
To fine tune this process, Sirimewan used guided segmentation to identify anomalies in mixed construction waste.
She is also working with fellow PhD students to trial the technology using robotic arms at the ASCII lab.
“We are continually refining our models for application in new robotic technologies and working closely with colleagues who are trialling it through the simulation of robotic arms,” she said.
Post-PhD, Sirimewan plans to focus on implementation of the technology, and is hoping these trails lead to investment in robotics and automation to improve the nation’s approach to construction waste processing and recycling.
“We are trying to ensure safety and health, not take away job opportunities,” she said. “In fact, it’s likely automation will create more skilled roles, because we still need workers to monitor the process and handle those machines and technologies.”