Visual semantics allow robots to recognise places from opposing viewpoints.
Place recognition is vital for robots and autonomous cars, but the task is a challenge.
For example, while humans can do a U-turn on the road and still recognise a place, robots and autonomous vehicles struggle to do the same.
Now researchers from Queensland University of Technology’s Science and Engineering Faculty and the Australian Centre for Robotic Vision have carried out what is believed to be a world first: using visual semantics to enable robots to recognise places from opposing viewpoints.
Current solutions use remote sensing such as LiDAR when it comes to autonomous cars, but Professor Michael Milford, Chief Investigator at the Australian Centre for Robotic Vision, says such systems don’t necessarily have a deeper understanding of the world around them.
“It doesn’t necessarily see that there’s a tree or a car or a pavement off to the side. It just sees objects without any particular understanding of what they are,” he said.
The QUT team instead drew inspiration from the ways humans perceive the world to create a more meaningful description of the environment and potentially change how users interact with autonomous vehicles.
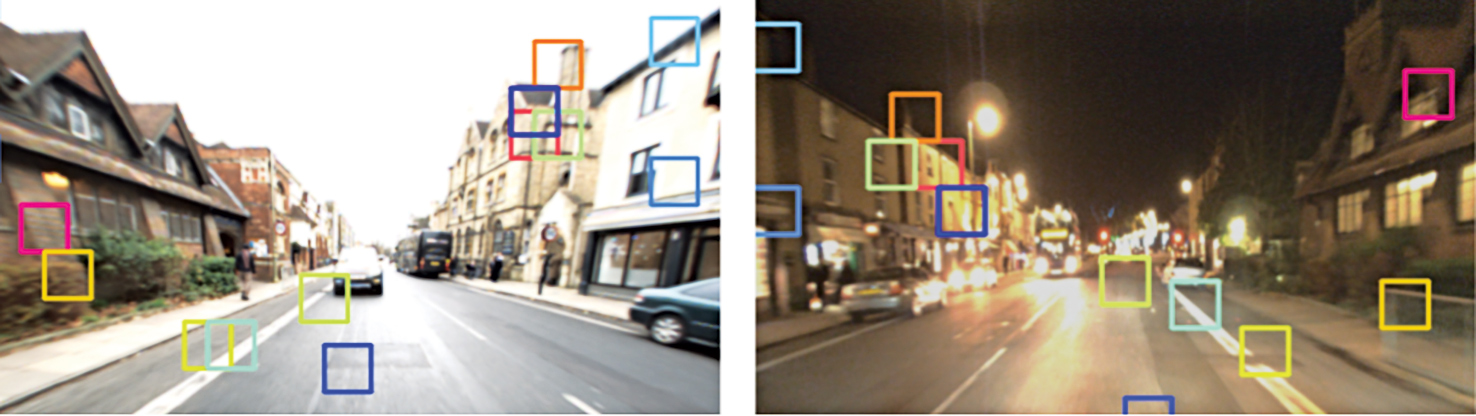
For example, a user might currently direct an autonomous car to a certain address on a map.
But a system that uses visual semantics would permit users to interact with it more naturally, giving a direction like, “drive down this road until we get to a park and then stop,” similar to how they would talk to a human driver.
The proposed system uses a semantic segmentation network called RefineNet. It was trained on the Cityscapes Dataset to form a Local Semantic Tensor (LoST) descriptor of images.
The team is also developing algorithms for autonomous vehicles in mining around navigation and positioning systems for underground trucks.
The team plans to continue developing this system’s capability to a product that could be rolled out on to an actual fleet of cars, or other domains like construction or mining.