As the capacity of machine learning grows, new challenges arrive with it. One engineer is finding better ways of dealing with shifting and imprecise data sets.
After working on decision support systems for her PhD, University of Technology Sydney (UTS) Distinguished Professor Jie Lu was certain a lot of work was needed on uncertainty issues.
After 20 years, her mind is unchanged.
Lu’s research and industrial projects are prolific, helping better decisions get made in scenarios ranging from customer churn prediction for telecom products, recommendation systems at Domain, and in identifying and creating a knowledge base for gene research with 23Strands.
Ever caught a Sydney Trains replacement bus due to weekend trackwork? You will have benefited from Lu’s wisdom.
Her team has helped crunch vast amounts of information to find patterns based on data sources, including from Opal card tap-ons and tap-offs, Google travel times, and the size of the bus fleet. This allows them to optimise timetables to keep waiting and travel times to a minimum and provide expected carriage loads along a train journey.
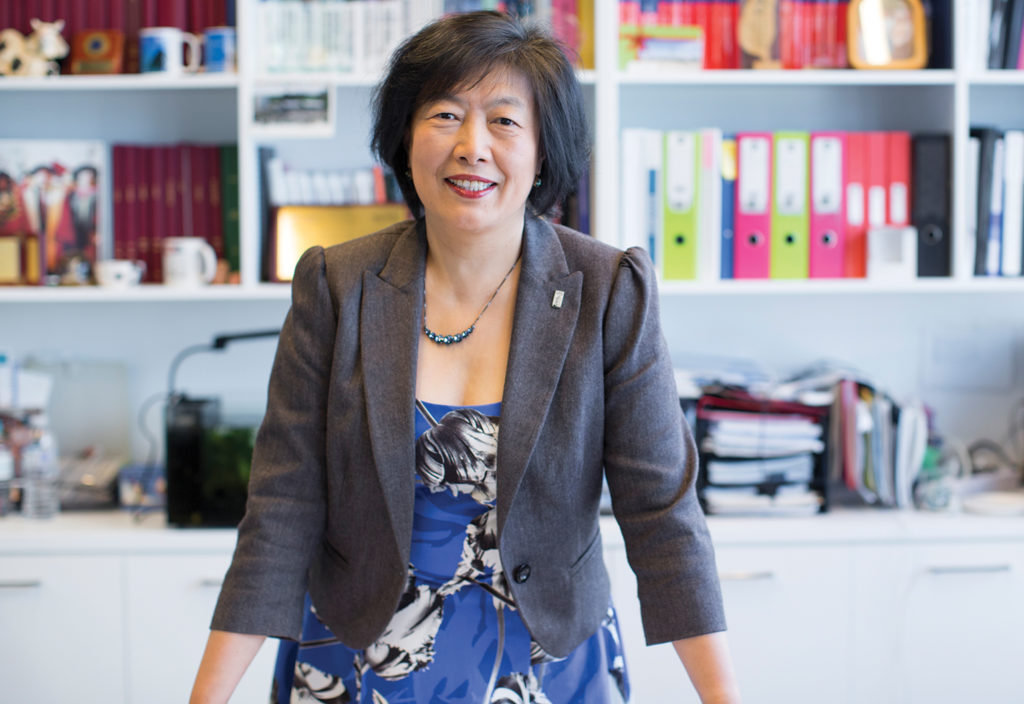
She has never questioned her decision to spend two decades and counting on computational intelligence problems.
There are just too many things to improve. Too many uncertainties acting on other uncertainties, waiting to be unpicked.
“For example, businesses have a very dynamic environment and a lot of missing data, and also quite a number of people use natural language to describe their business plans and goals,” Lu told create.
“So I have integrated fuzzy technologies into decision system modelling so that we can … handle various uncertainty issues to support business decision-making.”
Her development and application of “fuzzy transfer learning and machine learning-based decision support techniques” saw Lu named one of Australia’s Most Innovative Engineers by create in 2019.
“What’s really interesting about her work is she’s taken a problem and brought something entirely new to the space,” Professor Ian Burnett, Dean, Faculty of Engineering and Information Technology at UTS, told create.
“She’s taken these fuzzy models and applied them to a problem which has significantly improved the results that you get from a typical data prediction model.”
Data, models and more
Lu studied for an undergraduate degree in mathematics and a master’s in computer science before earning a PhD from Curtin University in information systems. Following that, she moved to UTS.
She splits the last two decades up neatly into a first half that focused mainly on model-driven decision support systems and a second on data-driven decision support systems.
The explosion in the amount of data created by businesses and other entities has driven this. Different kinds of machine-learning technologies are needed to make sense of this for prediction and decision support.
Lu’s past decade of work is further divided into two types of decision support situations: fast-changing data streams and “data insufficient” scenarios.
Industry is currently creating many streams of data to interpret — for example through the countless sensors that a business might use. This, along with other big data challenges, requires filtering through a lot of noise and drift to find useful patterns.
“If the data stream keeps changing very significantly, the model you use from past data developed doesn’t work, and that is a problem,” Lu said.
“So we developed algorithms to first identify drift. If there is drift, we can tell people and we can generate early warnings, and then we can adapt the model and we can support real-time decision-making.”
The second situation is one where, instead of a flood of data, there is too little on which to train machine-learning models.
Instead of building new models from scratch, which would be impossible, fuzzy transfer learning takes information from a similar source domain, such as movie recommendations, and applies it to a target domain, such as book recommendations.
What changes
The professor’s work has earned her fellowships with the International Fuzzy Systems Association (IFSA), the IEEE, and most recently as an Australian Research Council Australian Laureate.
The latter made her the first UTS academic to achieve the honour, with a five-year project on a new research direction in artificial intelligence (AI) beginning in January.
The work aims to develop new theories, methodologies and algorithms that can “give artificial intelligence the ability to learn autonomously from data” to support business decision-making in complex situations.
This year, the UTS Centre for Artificial Intelligence, which is directed by Lu, will also formally become the Australian AI Institute.
The institute, which comprises 200 staff and PhD students, formally launched on 7 August. Lu said its projects will include efforts to enhance AI with explanations to make solutions less like a black box — a description often used to describe machine-learning approaches.
Burnett — who nominated Lu for the 2019 Most Innovative list — describes his colleague as a rarity in contributing both senior leadership and a voluminous research output.
“And a role model for female engineers and computer scientists as well,” he said.
“She works really hard on mentoring and ensuring her postgraduates — and particularly women — succeed.”
Asked how her field will change life for engineers, computer scientists and others, Lu offers that systems that can learn on their own will transform the relationships between themselves and people.
She believes the news will be positive for engineers.
“I think basically the future of AI will promise significant improvement in engineering productivity and accuracy,” suggested Lu.
“I think for engineering that is very important, because the way people will create — and people’s skills — will be enhanced by speed and by the computation of complex situations.”
This article originally appeared as “Searching for certainty” in the August 2020 edition of create magazine.